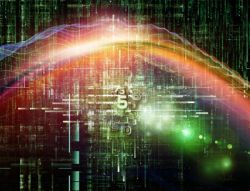
The importance of artificial intelligence has been increasing in many areas. A team of researchers led by Hans Briegel has now shown that quantum physics may help artificial intelligence agents to speed up decision making processes that are based on previous experience. This also provides a quadratic speed-up for active learning in situations where the agent actively explores its environment. The physicists have published their results in the journal Physical Review X.
Robots and autonomous machines are assuming an ever increasing range of tasks in our society. Future artificial agents need to be increasingly flexible and work intelligently by learning from previous experience, and they should be able to react autonomously to their environment. The more complex the environment the longer it takes the agent to compare a situation to previous experiences and come to a conclusion on how to respond. “Our agent model comes to a rational conclusion faster because, by using quantum physics, it is, in a manner of speaking, able to recall previous experiences simultaneously, searching for the best action,” reports physical theorist Hans Briegel from the Institute for Quantum Optics and Quantum Information of the Austrian Academy of Sciences and the Institute for Theoretical Physics of the University of Innsbruck. Together with a research group led by Miguel Angel Martin-Delgado from the Institute for Theoretical Physics at the Complutense University in Madrid, Hans Briegel’s research group developed an AI model that uses this form of quantum parallelism.
Projective simulation
Two years ago Hans Briegel suggested a model for an artificial intelligence (AI) agent that perceives percepts from the environment, processes these percepts in a specific type of memory which allows it to internally simulate future possible actions. The episodic and compositional memory (ECM) consists of a network of clips, which are fragments of experiences. When the AI agent is confronted with a new event, it starts performing randomized searches of related previous events, using a process called (quantum) walks. The path through the memory is determined by transition probabilities modified according to previous experience. In this way the AI agent learns from success and failure and is able to create new clips that are then integrated as if they were previously encountered percept-action events. This provides the agent with a platform for projecting itself into simulated situations. The scientists have now combined this concept of projective simulation with the principles of quantum information processing. “A random walk is substituted by a quantum stochastic process, which allows for a more efficient exploration of the memorized experiences,” explains junior scientist Vedran Dunjko. “This is why the quantum agent is considerably faster or to be more precise, quadratically faster in terms of taking action than a conventional agent.” This difference can be vital for many applications, for example when one thinks about the hot topic of self-driving cars.
Active Learning
The speed of the agent‘s internal decision-making process is particularly important for active learning. Robots that operate in an unknown environment, which may also vary over time, explore it in their own speed. Thus, faster operating agents are better prepared to act in quickly changing environments, and they have more time to try out new courses of action and develop successful patterns of behaviour. The application of quantum physical methods to problems of artificial intelligence is still in its infancy, underlines Hans Briegel. “However, studying and using autonomous and active learning systems in the context of quantum experiments could steer research into entirely new and exciting directions.”
The research was financially supported by the Austrian Science Fund FWF and the American Templeton Foundation.